Forward:
On a recent call with Elias Gröndal, Amy Washington and myself, we got into a debate between the ideal structure of a product hierarchy - this article comes off the back that heated and passionate call #nerds.
For those who don’t know; Elias the co-founder of Resourced - a PLM solution used by some of the best in luxury fashion - before co-founding Resourced, Elias spent 8 years at H&M in various roles across product and supply chain.
Amy recently joined the Commerce Thinking team following a successful career running merchandising and product teams at brands like; Burberry, Acne, Reiss, Allsaints and more…
So, it’s safe to say that these two know their sh*t when it comes to product and hierarchy. Without further ado, here’s the debate in full copy-written form.
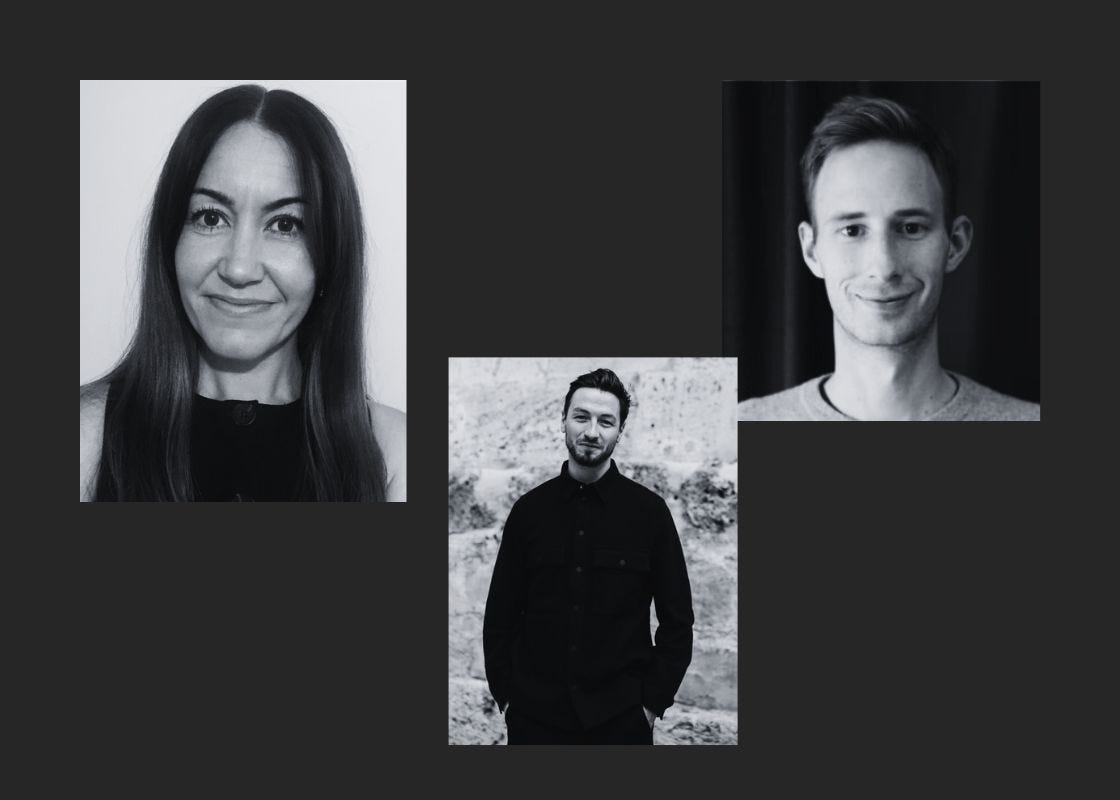
Hierarchy vs. Attributes
Retail brands today are no strangers to the tug-of-war between structure and flexibility. On one side, there’s the classic product hierarchy—a tried-and-true way to keep things organised, offering broad categories that bring a semblance of order. But let’s face it: a rigid hierarchy can become more of a straightjacket than a guide, especially when your brand needs to stay adaptable. That’s where attributes come in. Attributes offer the freedom to layer on specifics—“occasion,” “material,” “style”—without permanently locking products into set categories. But can brands really have the best of both worlds?
In this article, we’ll unpack how forward-thinking brands can balance these two approaches, embracing the structure of a lean hierarchy while allowing attributes to do the heavy lifting for adaptability. Yes, hierarchies serve a purpose, but as retail evolves, there’s value in questioning long-held practices and rethinking how data should flow across the business.
A more flexible, cross-functional data model won’t just save time; it’ll free teams up to work strategically rather than fixating on where products “should” sit. Whether you’re scaling product lines, adapting to new trends, or simply looking to streamline operations, finding the right balance between hierarchy and attributes could be the key to a more agile, responsive brand.
The Pros and Cons of Hierarchical Data Structures
The product hierarchy is a staple of retail data management, and for good reason. Hierarchies give structure to sprawling inventories, providing broad categories that create order across a brand’s various departments. From “outerwear” to “accessories,” these high-level groupings make it easier for teams to classify and manage products at scale, guiding everything from budgeting to stock allocation. But while a hierarchy offers simplicity, it often comes with hidden limitations—especially when a brand needs to remain flexible and responsive to change.
What Hierarchies Offer
At its core, a hierarchy offers stability. By setting up clear, consistent top-level categories, brands create a system that’s easy to manage and reference. Departments across the business can use the same baseline categories, enabling smoother data flow and alignment between teams. For example, merchandising and buying teams can rely on a shared “category” framework to make budgeting decisions and inventory projections. A strong hierarchy helps brands allocate resources more efficiently and maintain consistency, even as product ranges expand.
Where Hierarchies Fall Short
But hierarchies can quickly start to feel like a straitjacket. Because they’re built around fixed categories, hierarchies can struggle to accommodate the nuances of modern retail—whether it’s genderless clothing, seasonal product lines, or rapidly evolving trends. This rigidity often leaves brands stuck with categories that may have made sense years ago but no longer reflect the current market.
Take, for example, the rise of unisex or “gender-neutral” apparel. Traditional hierarchies often force products into male or female categories, leading to workarounds that can complicate data management and confuse customers. Or consider a brand that wants to introduce a new product category mid-season. With a rigid hierarchy, teams might find themselves jumping through hoops to make the new category fit within an outdated structure, wasting valuable time on reorganisation rather than innovation.
As a result, teams often become “precious” about maintaining the hierarchy, resisting change for fear of disrupting established processes. But in today’s market, clinging to rigid structures could mean missing out on valuable opportunities to adapt. Instead of being overly protective of the hierarchy, brands can benefit by keeping it lean, treating it as a flexible framework rather than a fixed set of rules.
Attributes as a Modern Solution to Product Data Flexibility
If hierarchies provide structure, then attributes offer freedom. Attributes allow brands to add depth and detail to products without permanently locking them into specific categories. Think of them as adaptable labels, capable of capturing the unique qualities that differentiate each product—qualities that don’t always fit neatly within a rigid hierarchy.
What Attributes Bring to the Table
Attributes are especially valuable in today’s fast-paced retail environment, where flexibility is essential. Instead of creating countless subcategories within a hierarchy, brands can use attributes to tag products with specifics like “occasion,” “material,” “colour,” or “fit.” This approach keeps the hierarchy lean, while providing the extra information teams need to classify, sort, and filter products in ways that adapt to changing trends and customer needs.
For instance, an “occasion” attribute can tell e-commerce teams which products to highlight for seasonal promotions—like a “winter holiday” or “summer casual” look—without disrupting the core structure of the product hierarchy. Similarly, a “fabric” attribute allows merchandising teams to quickly identify all products made from a specific material, like organic cotton or recycled polyester, across multiple categories. By focusing on attributes, brands create a flexible layer of detail that can be easily adjusted as trends evolve, without needing to overhaul the entire system.
The Risk of Overusing Attributes
However, attributes are not a free-for-all. While they offer flexibility, overloading a product with too many attributes can lead to inconsistency, confusion, and even errors. When every department adds its own attributes without a cohesive strategy, the data can quickly become fragmented. A high level of detail is valuable, but if attributes aren’t standardised, or if they’re used without clear guidelines, data entry can become subjective. This inconsistency can make it difficult to pull reliable reports, analyse data, or ensure accuracy across teams.
To prevent “attribute overload,” brands need a clear, collaborative approach. Establishing a shared attribute “playbook” helps keep definitions consistent, ensuring everyone understands when and how to apply each attribute. This playbook doesn’t just prevent redundant or conflicting tags; it also makes it easier for departments to trust and use product data, no matter where it originates.
Embracing Flexibility While Maintaining Control
Attributes are not a replacement for hierarchy but a complement. While the hierarchy provides a stable foundation, attributes offer the flexibility to add depth and nuance. When used thoughtfully, attributes allow brands to be responsive and innovative, without sacrificing control over data quality.
Real-World Examples of Balanced Data Models
A = Category-Led Hierarchy with Attributes
This model is all about classic organisation, mirroring how buyers and merchandising teams traditionally operate. It starts with high-level categories like brand concept (e.g., own brand or branded collections) and is further refined by gender divisions (mens, womens, unisex) before diving into specific product categories (dresses, knitwear, shoes, etc.).
Attributes like fabric, fit, or occasion add depth, offering flexibility to highlight nuances without disrupting the core structure. Think of this as a stable framework that keeps things neat but leaves room for detail and customisation where it counts. Perfect for brands where top-level categories guide decision-making and collaboration.
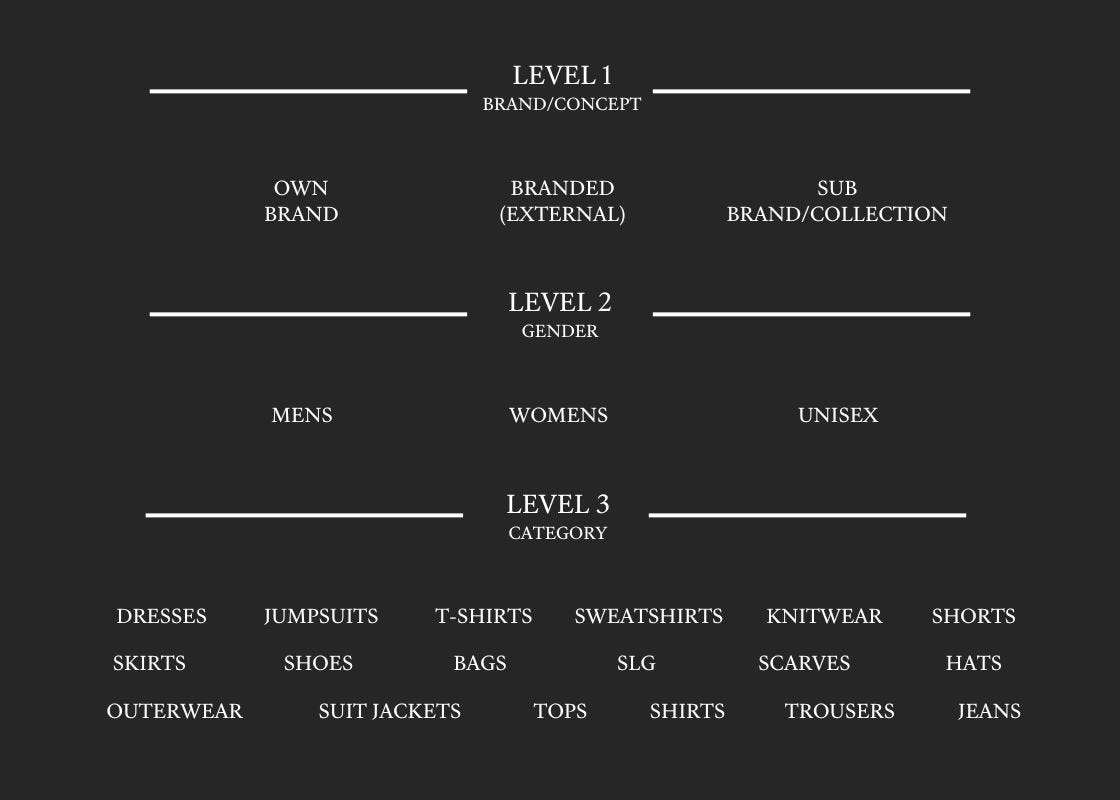
Attributes could include things like:
Sub-Category (Jackets, Coats, Leather Jackets, Quilted Jacket, Down Coat…Hoody, Sweatshirt, Zip through…Sweater, Cardigan, Vest…Cap, Beanine, Bucket hat…)
Season (AW24, SS25, AW25…)
Collection (NOOS, Carry Over, Seaonal..)
Length (Midi, Maxi, Short, Regular, Long…)
Fabric (Wool, Cashmere, Leather, Denim…)
End Use (Day, Occasion, Casual, Formal…)
Print (Printed, Plain, Spot, Animal, Stripe, Check…)
Fit (Oversize, Regular, Fitted…)
Shape (Wide leg, Barrel leg, Skinny, Straight…A line, Bodycon, Empire, Wrap, Shift…)
B = Product-Type-Led Hierarchy with Attributes
For those who favour simplicity and function, this structure organises products by type—grouping jackets, shirts, footwear, and accessories into clear buckets. It’s straightforward and easy to navigate, with attributes like sleeve length, fabric type, and sustainability certifications adding the extra detail needed for more precise sorting and filtering.
This approach is a no-nonsense model designed for operational efficiency, helping teams quickly identify and manage similar items while relying on attributes to handle the specifics. Ideal for brands focused on streamlining processes and cutting down on complexity.
Attributes could include things like:
Sleeve length (Only for Apparel with product type having sleeves)
Fit (only for apparel, product types for upper body)
Fit (only for trousers)
Waistline (only for trousers)
Neckline (Only for Apparel with product type having sleeves)
Type (Apparel & Accessories)
Process (Footwear)
Sustainability (All)
Collection (All)
Functional (All)
C = No Hierarchy
The wildcard of the bunch, this model does away with a fixed hierarchy entirely, instead embracing a fully attribute-driven structure. Every product is tagged with detailed attributes—fit, fabric, season, use case—allowing for maximum flexibility and adaptability.
This is the ultimate solution for brands that want to break free from traditional constraints and build their data model around evolving needs. It’s agile, future-proof, and perfect for teams who prefer innovation over convention.
Practical Steps for Implementing a Balanced Data Model
Achieving a balance between hierarchy and attributes isn’t a one-size-fits-all process. Each brand has unique data needs, and finding the right balance requires a thoughtful approach. By focusing on simplicity, consistency, and collaboration, brands can build a flexible data model that supports agility without sacrificing control. Here’s a practical guide to help your brand implement a balanced data model:
1. Define a Lean Hierarchy with Essential Categories Only
Start by mapping out the high-level categories that will form your core hierarchy. Think broadly—product type, category, and perhaps gender if necessary. These foundational levels provide a stable structure without the need for overly specific, hard-to-manage subcategories. Keeping your hierarchy lean makes it easier to adapt as new trends emerge, preventing the frustration of constantly adjusting rigid categories.
For example, instead of defining specific seasonal categories like “spring jackets” or “summer dresses,” use broad categories such as “outerwear” or “apparel” and leave room for more adaptable classifications at the attribute level. This approach lets your hierarchy provide order while allowing attributes to capture the details that change over time.
2. Standardise and Limit Attributes for Consistency
Attributes offer flexibility, but without a clear strategy, they can easily become unwieldy. To avoid “attribute overload,” select only the most essential attributes that each team needs to function effectively. Examples include “season,” “occasion,” “fabric,” and “fit.” These can be valuable across e-commerce, merchandising, and product development without cluttering the data model.
Develop a shared “attribute playbook” that standardises attribute definitions, spelling, and usage rules. This playbook becomes a reference point, ensuring that all teams tag products consistently and in ways that align with overall business goals. By limiting and standardising attributes, you create a flexible layer of detail that’s manageable, accurate, and easy to use.
3. Automate Data Flow to Minimise Manual Entry
Automation can help reduce the time teams spend on low-value, repetitive data tasks and limit the risk of human error. Integrate tools to sync your product data across key systems, such as PLMs, ERPs, and e-commerce platforms. This approach ensures updates are automatically reflected across departments without needing manual re-entry.
For instance, automating data flow from the PLM to the e-commerce platform eliminates the need for e-commerce teams to constantly reformat and adjust product data. Automation also helps enforce your data model by ensuring fields stay consistent, removing the need for manual cross-checks and adjustments at each stage.
4. Establish Regular Data Checkpoints
Regular data checkpoints help catch any inconsistencies and keep both hierarchies and attributes aligned. Schedule data audits at key points in the product lifecycle—such as product launch prep, seasonal transitions, or inventory planning periods—to identify any discrepancies or mismatches in the hierarchy and attributes.
These checkpoints can be as simple as spot-checking records or more in-depth, automated validations. By auditing data consistently, brands can maintain data quality, prevent errors from accumulating, and ensure that all teams have accurate, up-to-date information to work with.
5. Create a Cross-Functional Data Squad
Establishing a cross-functional data team—or “data squad”—can help maintain alignment across departments. This team, with representatives from e-commerce, merchandising, product development, and marketing, collaborates on any necessary updates or changes to the hierarchy and attributes. Together, they ensure that changes are well-communicated and that all teams understand the implications.
This squad doesn’t just help with initial setup; they play an ongoing role, periodically reviewing and optimising the data model to keep it aligned with business goals and market changes. By making data management a shared responsibility, brands reduce the risk of silos and build a data model that remains relevant and adaptable.
Putting It All Together
A balanced data model is the foundation of an agile brand. By keeping hierarchies lean, attributes standardised, and automation in place, brands create a streamlined data structure that’s easier to manage, adapt, and optimise. Through regular data checkpoints and cross-functional collaboration, this approach ensures your data model can support both current operations and future growth—freeing teams from “busywork” and empowering them to focus on work that truly drives value.
If your brand is struggling to find the sweet spot between rigid hierarchies and freeform attributes—or if you’re unsure how to create a balanced data model that supports agility and innovation—we’re here to help.
Whether you're refining your existing structure or starting from scratch, we can guide you in building a hierarchy and attribute strategy tailored to your business needs. Book a consultation call today to simplify your data, improve cross-functional collaboration, and future-proof your operations.